An analysis of 100+ variables around VC funds, paired with performance data, surfaces dozens of correlations that can help LPs improve their manager selection process.
‘How do we pick the right managers in venture capital?’ is a question of perpetual concern to every LP. The answer can make or break portfolios and careers.
Many LPs have a strong conviction about a particular approach to evaluating and selecting prospective venture investments. Some LPs are incredibly data driven. Some lean into industries they intimately know. Others focus entirely on the individual people behind the firm. Still others hunt for uncharted waters and arbitrage opportunities.
Frequently, LPs default to a ‘pattern match’. The problem is whether the pattern is any good.
Today, the most common ‘pattern match’ that LPs apply to venture capital selection is this:
- Ivy League graduate,
- Spin-out from another (preferably name-brand) VC firm,
- With a prior attributable track record.
- Throw in varsity athlete, and you’ve got a winner (yes, we’re not kidding…a surprising number of LPs think this is predictive).
But patterns — if they even work — can certainly change. And in an industry driven by innovation, those who think and look different may be best poised to capitalize on the next big thing.
The Evidence Contradicts Conventional Wisdom
Many have seen the growing body of evidence that shows first-time funds and smaller funds outperform. Cambridge Associates have published a host of research on this. We’ve been tracking similar metrics. Some institutional LPs have learned from this and created emerging manager programs to build relationships with these managers early. But many LPs default to the usual suspects, as shown in part by record capital flows to established managers and mega funds.
Arguments against emerging VCs include the highly dispersive nature of returns and the sheer challenge that comes with navigating hundreds of potential emerging managers. They find it difficult to underwrite risk or project performance. By our metrics, there are ~260 U.S.-based VC firms on their 4th fund or later (thus showing ‘full’ track records’), but ~1,250 firms considered to be emerging. Finding the right small funds is no small feat.
This can make selecting new managers — especially those on first-time funds — incredibly difficult. How do you pick when you can’t point to a track record? As a result, some LPs still default to the classic pattern match or eschew emerging managers entirely.
Alternative Performance Indicators: Predicting Outperformance
We believe that data can predict under- and out-performance, and uncover new and better patterns for selecting VC funds. With access to the right venture characteristics, we think LPs can navigate first-time funds and the emerging manager ecosystem with confidence.
At Different, we have built the most robust database on venture capital, tracking 100+ variables across thousands of firms, funds, and individual partners. Over the last year, we’ve begun analyzing these variables against IRR, TVPI and MOIC for hundreds of funds. While the model is early and a work-in-progress, the initial results shatter conventional wisdom around venture.
While no single variable stands on its own, there are dozens that have notable correlations for either outperformance or underperformance. And while there will always be exceptions and outliers — at the portfolio level — these indicators can help LPs screen in more talented managers and screen out more duds.
We’ve decided to publish a select few.
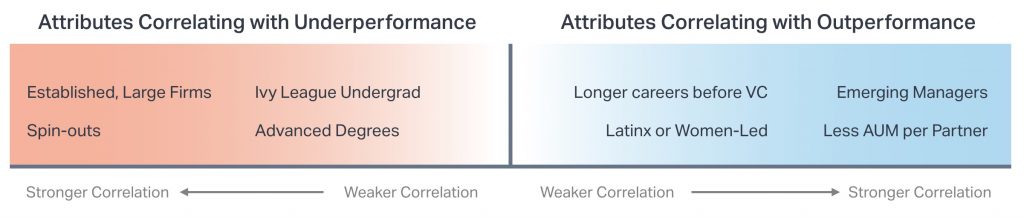
Our model shows that core components of the classic pattern-match — the Ivy League spin-out — correlate with underperformance. In contrast, VCs that tend to struggle to raise capital such as those led by women and Latinx GPs correlate with better returns. But this is just the tip of the iceberg: our full models and the specific degrees of correlation, along with our wide range of proprietary datasets, inform our work with our clients. Reach out to us if you’d like to discuss further.
Breaking the Patterns, Leaning into Opportunities
Naturally, the data is full of nuance. These are just a few of the 100+ variables we are examining. We are testing VC characteristics such as thesis, geography, ESG and impact, partner experience, fields of study, industry-expertise, and much more. We’ll continue to test and improve our models over time. We also recognize that what matters today may change tomorrow.
As we go forward, we’re excited to shed greater light on what really matters in venture capital. The future of venture capital can look Different.